Data Rights and Collective Needs: A New Framework for Social Protection in a Digitized World
Mariana Valente & Nathalie Fragoso
All social programs employ some ‘legibility’ scheme, to make citizens visible, readable, and verifiable to the state. Today, this trait is combined and enhanced by the datafication process. Social protection systems around the world are becoming increasingly computerized and reliant on beneficiaries’ data for related decision-making. Digital technologies that are capable of collecting and verifying large amounts of data are employed to this end, impacting the exercise of both digital and social rights. In this essay, we will address the differential impacts of the datafication of social protection on marginalized populations, using examples from existing literature and our own research. We then engage with existing reflections on social protection and datafication to highlight the importance of a data justice framework for the current global political and economic context.
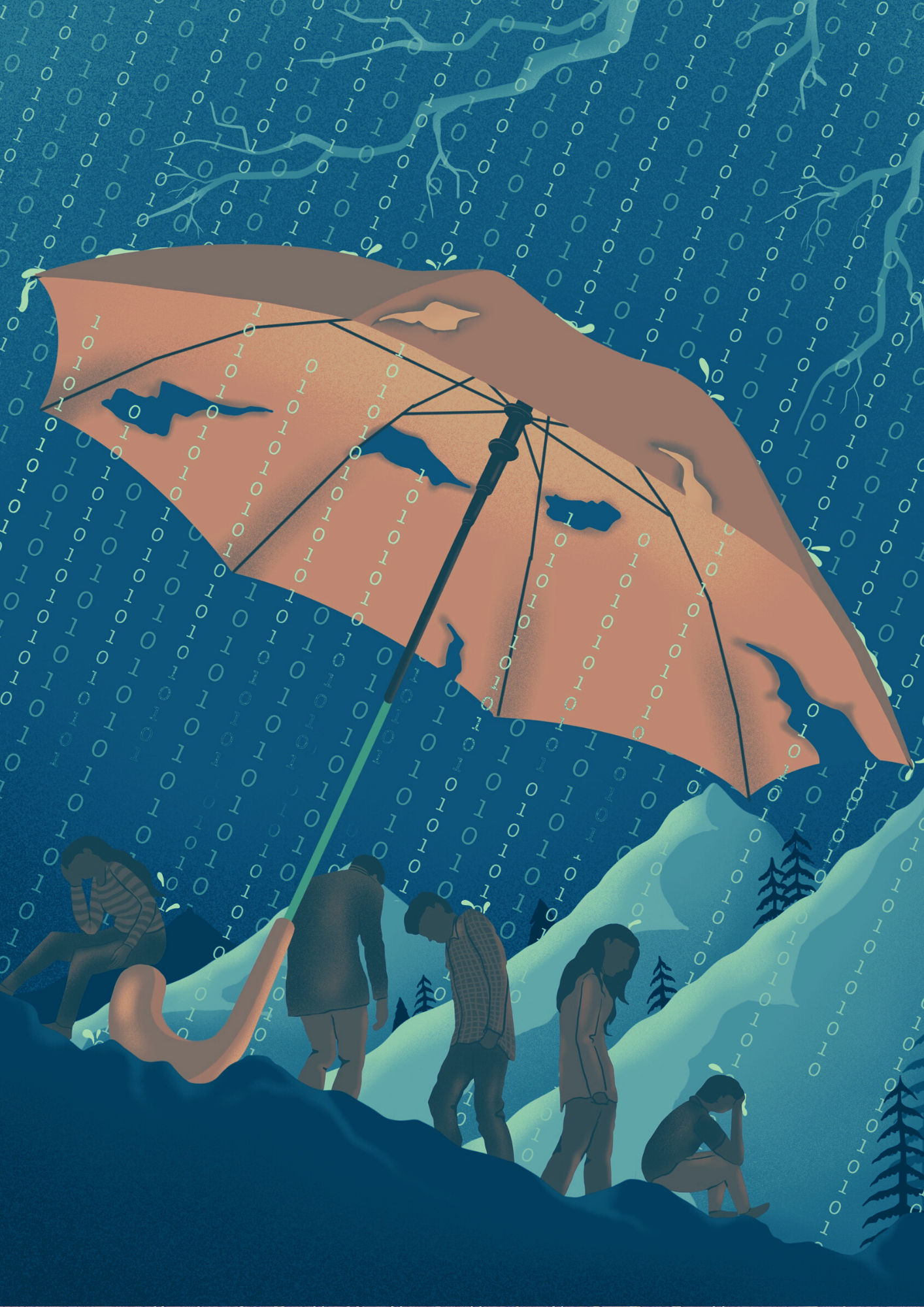
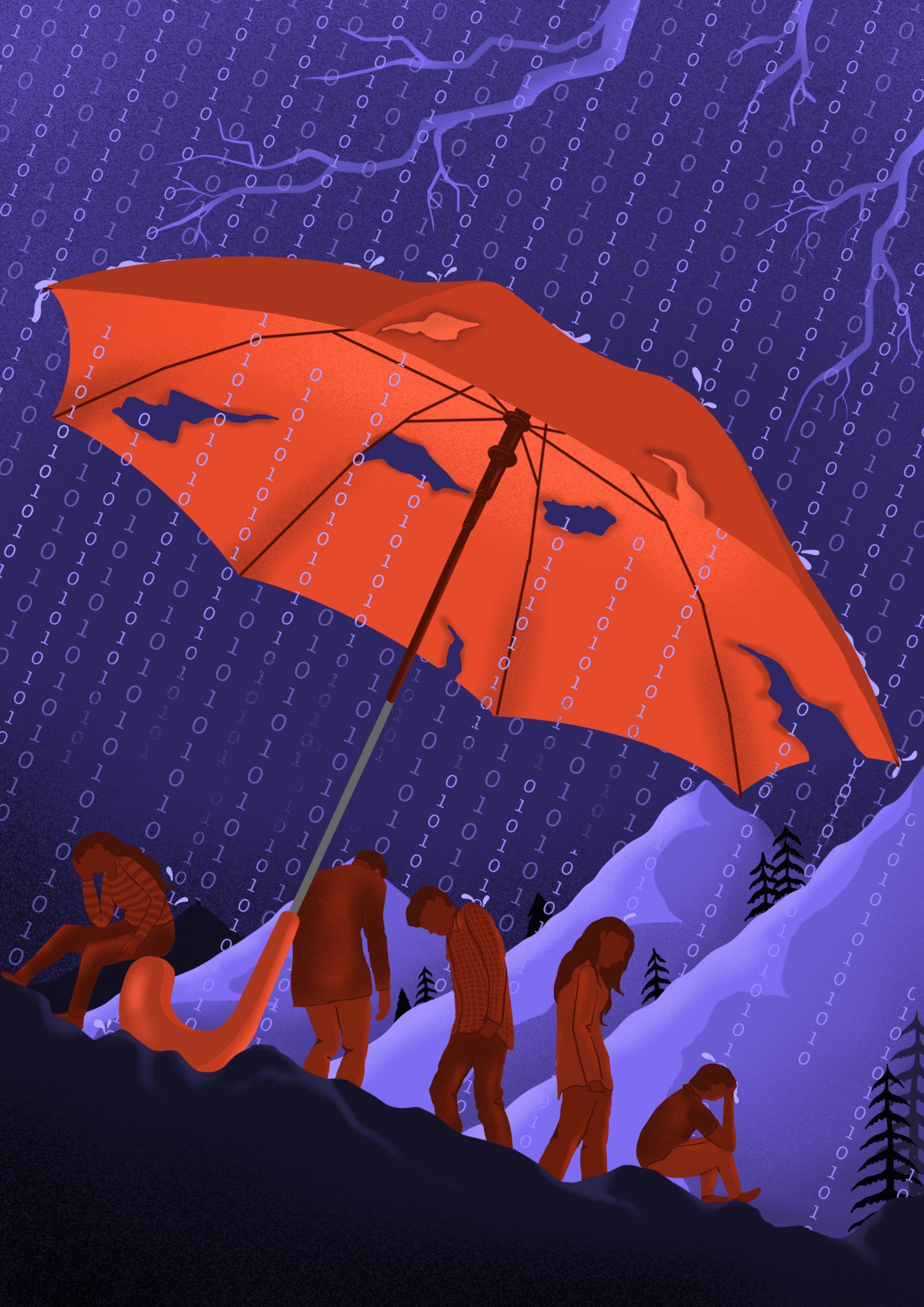
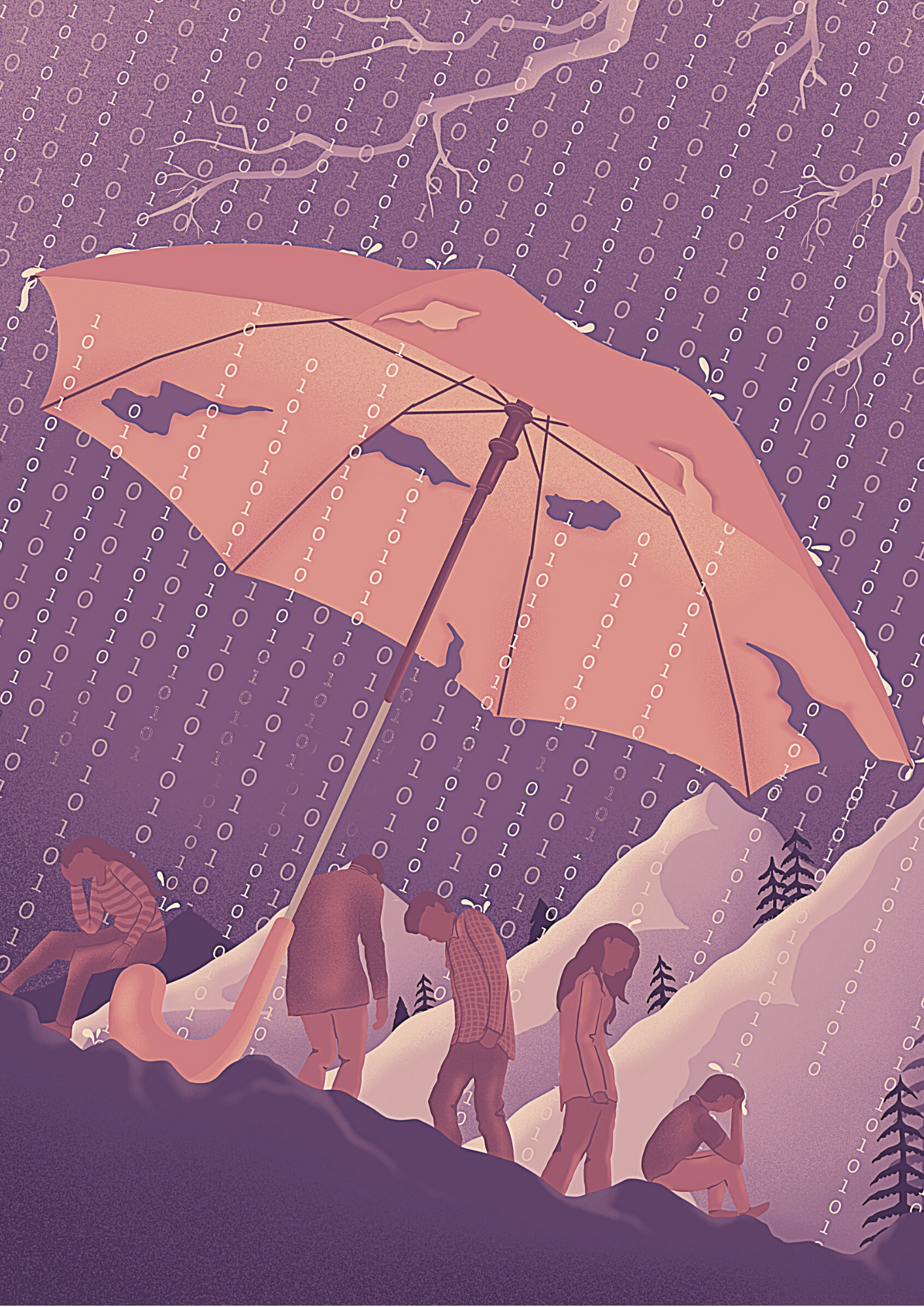
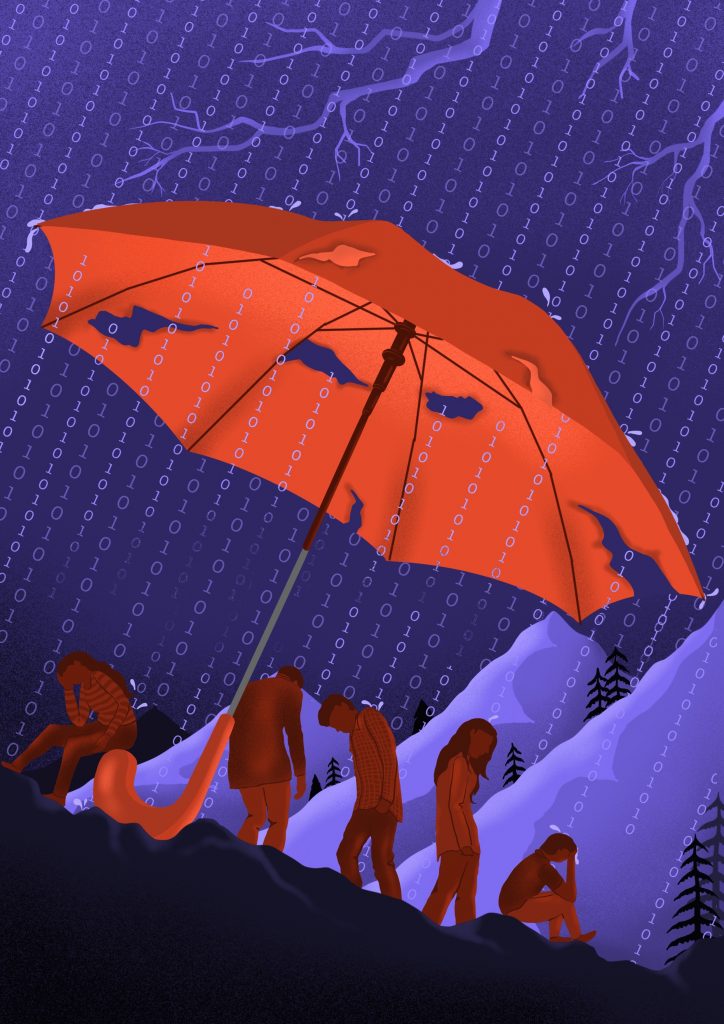
Introduction
Sixteen-year-old Ana, who belongs to a minority religious and ethnic group, was forced to flee her home in a Latin American country when violent conflict broke out. She and her family crossed the border into a neighboring country in the Americas. Once there, her parents applied for refugee status and social protection benefits and waited for an official response.
After a six-month wait, law enforcement officials visited their home – which they share with another immigrant family – armed with a database of all asylum applications, with details like the number of accompanying children, ethnicity, and religion of the applicants. Typically, this data is crossed-checked against additional information obtained from social media handles and biometrics collected at the border for intelligence purposes. This task is performed by a private company that is in partnership with another (also private) corporation that specializes in facial recognition technologies, and to this end, owns and manages a database of images of over 300 million citizens worldwide.
When Ana’s face was scanned at the border as part of immigration procedures, the system could not find her in the database – although it did find her brother Luiz, a successful gamer with a substantial online presence. But now, Ana’s face is also part of the database that, among other things, is used by the police for crime-solving. Her facial features are recorded and linked to her fingerprints, her name, and other identifiable information. This is the same database that, according to a media report, law enforcement officials relied on to ‘mistakenly’ arrest a black man when the facial recognition system tied to the database returned a false positive.
To an extent, Ana is aware that data about her is being collected and stored – she had to answer several questions posed by multiple border officials. But she does not know the details of how this information will be treated, and whether or how she can access it. She is not aware, for instance, that the data thus collected is shared with multiple government agencies and private stakeholders, or that her social media information is being collected and used. She has no idea that the biometric data collected from her can be used for crime investigations. She does not know that people who have not come in contact with immigration and social protection services are less represented in all these databases. All she knows is that this information will be used by the government to decide whether her family is eligible for a conditional cash transfer program; it will determine her immigration status and her ability to go to school in the country where she now lives.
Ana’s situation is hypothetical but based on real-life incidents. It raises many questions about data, digital technologies, and access to social protection programs. In this essay we are concerned with what happens to social protection programs when they become datafied.
In Seeing Like a State (1998), J. Scott introduces the concept of ‘legibility’ to analyze how states use information about their citizens to achieve certain goals. He outlines a process in which states simplify and standardize citizens’ data for purposes of social control, thereby dissolving local and contextualized understandings. Signifiers and measures which are ubiquitous today, were historically, more often than not, created and enforced by modern states. Permanent surnames, for instance, were almost everywhere a state project to fix an individual’s identity, link them to a group, and promote the status of male family heads. They were useful for taxation, property rolls, and censuses. Cadastral mapping of land holdings and standardized weights and measures were part of elaborate and costly state campaigns. These attempts to classify and assimilate – aimed at making objects ‘legible’ to the state – were often met with localized and grassroots resistance. They stood in direct opposition to local practices which were multiple and diverse, and served a community that understood them.
Legibility also implies that things are overly simplified. In contemporary societies, the complexities of social life are necessarily flattened out, for instance, when states classify citizens’ economic situations into income tax bands. To be sure, some such standards are necessary for centralized planning and monitoring – and colonial powers have employed them extensively. However, such categorization also has a direct impact on how legal and administrative measures apply to people and situations, and they also, in turn, shape reality (Scott, 1998).
All social programs make use of some legibility scheme. They aim to make citizens visible, readable, and verifiable to the state by putting them into simplified and standardized categories.
All social programs make use of some legibility scheme. They aim to make citizens visible, readable, and verifiable to the state by putting them into simplified and standardized categories. Cash transfer programs, for instance, reduce the complexity of individual situations in a given territory to the category of ‘poverty’ or ‘extreme poverty’. Similarly, digital technologies that are capable of collecting and cross-checking large amounts of data relate, at different levels, to legibility, visibility, and readability. The treatment of this data, which frequently takes the form of big data, is often conducted by third parties: private sector, academic, or non-profit institutions.
As Linnet Taylor and Dennis Broeders argue, an earlier landscape characterized by “data for development” collected and treated primarily by the state, is now being replaced by a “messier, more distributed landscape of governance where power accrues to those who hold the most data” – these are largely private entities. In this new landscape, the authors point out, citizens are frequently unaware of the data that they are providing to these entities. And perversely, citizens are also being made visible or legible by data of unknown (or questionable) reliability, biased by conditions such as internet connectivity or previous exposure to specific policies. This means that their ability to access social protection programs is tied to data that may be unreliable, collected without prior consent, and often under the control of private agencies. These factors, the authors argue, should compel us to look beyond the framework of legibility.
Linnet Taylor defines data justice as “justice in the way people become visible, represented, and treated as a result of the production of digital data”. It is a framework for data revolution that goes beyond a merely technical approach to one driven by a social justice agenda.
In this context, a growing body of literature, especially in development studies, has been looking at people’s interactions with digital data through the perspective of data justice – or data injustice, for that matter. Taylor (2017) defines data justice as “justice in the way people become visible, represented, and treated as a result of the production of digital data”. It is a framework for data revolution that goes beyond a merely technical approach to one driven by a social justice agenda.
Data justice is better understood when tied to a sister diagnosis – that of ‘datafication’. According to Heeks and Shekhar (2017), datafication can be defined as the increasing use and impact of data on social life. When processes that relate to and are conducted by people, become increasingly computerized and reliant on data, we can say that a process of datafication is underway. When it comes to social protection or development policies and their increasing use of technology, we might also be speaking of data being made available on populations that had hitherto been digitally invisible.
Visibility, however, can be ambiguous. While some argue that it is central to de-bureaucratization, modernization, and citizenship consolidation (Kanashiro, 2011), visibility can also create new risks and exaggerate particular power imbalances. People subjected to various kinds of social stigma, groups that are routinely discriminated against, and citizens who bear the status of informality or illegality, may be justifiably fearful of becoming visible – whether digitally or otherwise.
Datafication requires a data justice perspective because its potentially negative consequences are felt most severely by those who are already disadvantaged by existing inequalities along the lines of race, class, gender, socio-economic status, and other social markers. The datafication of social protection, in particular, needs a data justice approach so that it is grounded in principles of social justice.
In this essay, we will address the differential impact of datafication on marginalized populations by drawing on examples from existing literature and our own research. Next, we will engage with existing reflections on social protection and datafication to highlight the importance of a data justice framework for the current global political and economic context.
1. When data decides
The Brazilian Bolsa Família Program is the world’s largest conditional cash transfer program. As of June 2020, it covered over 14 million families (or 43 million people) in poverty and extreme poverty. Although the program was introduced with the objective of providing social security as a universal right, it was gradually accepted as a focalized program – targeted at sections of the population that are understood as being the most in need. Over time, it was co-opted as a hegemonic welfare model, aligning with the guidelines of the World Bank and the Inter-American Development Bank (IDB), and furthering the neo-liberal project of successive Brazilian governments of the 1990s. Besides, it is a conditional cash transfer program linked to certain education and health outcomes: for instance, children must be enrolled in school, have regular attendance, and be given all the necessary vaccinations. Beneficiaries continue to be covered by the program only if they remain compliant. It is worth noting that, although by law the benefit program is meant for the family, it is provided preferentially to women who are more than 90 percent of its beneficiaries.
The selection of families for the program is automated, based on data stored in the Single Registry (CadÚnico). This federal registry informs all federal programs aimed at the low-income population, except for pension schemes. As of May 2020, 35 percent of the Brazilian population was part of the Single Registry. The extent of this database, both in terms of the number of citizens and the amount of data available on them, is aimed at finding vulnerabilities and fighting multidimensional poverty. It allows for the identification and assistance of populations to be targeted by public policies on basic sanitation, employment, and housing.
Data from the Registry is also shared between the agencies running the Bolsa Família Program and the ministries of health and education that provide data on school attendance and health duties relating to children in the family. The person responsible for the family unit is required to provide 77 different pieces of information for the Single Registry, with varying degrees of detail and sensitivity. There are training manuals to guide the interviewer, but nothing in them relate to data rights or informational self-determination. The interviewer is asked to make the beneficiary’s responsibilities clear, including providing factual information under penalty of criminal liability and maintaining up-to-date information, but there is no clarity provided on the state’s obligations with respect to the data thus collected.
Nowhere is it mentioned, for instance, that public service concessionaires, which are private companies, get access to the full Single Registry database, purportedly for informing programs on tariff benefits. In the past, this vast repository of data, evidently of interest to third parties with commercial, electoral, and social control interests, has been compromised several times, leading to substantial damage. On a few different occasions, beneficiaries received WhatsApp messages promising new benefits, which turned out to be a scam and and introduced some kind of malware into their cellphones, or they were directly reached by electoral campaigns.
It is also not mentioned that a beneficiary’s name, social ID number, and the amounts received as social benefit are published online for the sake of transparency. Our research shows that this, together with the incentives offered by the government to report fraud, has enabled a sort of social surveillance that takes on a gendered form. Women, who form a large majority of the beneficiaries, are stigmatized and reported for not spending their money on what is expected – the household and children.1
The datafication of social protection in Brazil has allowed for an increased legibility of vulnerable populations while also re-entrenching such vulnerabilities through increased data sharing among state organs, insufficient access control, austerity programs, and heightened state surveillance.
Focalization as well as verification procedures, linked to conditions for receiving benefits, are indeed constitutive features of the program. From its very inception, the Bolsa Família Program anchored its legitimacy in the efficiency and cost-effectiveness of the permanent efforts designed to target those who need it the most. These characteristics of the program have been accentuated in the past few years. Social and political shifts have created new political majorities and a concrete trend of social welfare cuts, which materialized in the form of budgetary and financial restrictions in the New Tax Regime (EC 95/2016). Recent decrees (for example, Decree 10.046/2019) also facilitate data sharing for detecting fraudulent and undue benefit claims. In this context, inclusion errors in social programs have gained centrality in the public sphere – and beneficiaries’ data is shared extensively across operations for general purposes as well as for specific investigations. In her Master’s thesis, researcher Isabele Bachtold concludes that these processes lead to “constant surveillance” and “a daily struggle to prove to be poor”. To this we would add the daily struggle to continually prove oneself deserving of benefits.2 In these ways, the datafication of social protection in Brazil has allowed for an increased legibility of vulnerable populations while also re-entrenching such vulnerabilities through increased data sharing among state organs, insufficient access control, austerity programs, and heightened state surveillance.
Experiences in other countries allow us to observe other consequences of datafication. In India, the biometric database Aadhaar, launched in 2009, has over a billion records and was created under the justification of allowing easier access to welfare by the target population as well as combating welfare fraud. Under the program, people below the poverty line need to confirm their identity through iris or fingerprint scans when collecting benefits. There is, however, plenty of documented evidence on how the system produces further injustices. There have been cases in which people could not have their fingerprints or irises read due to hard work or malnutrition, as fingerprints degrade or disappear. Besides, the requirement that only one family member be scanned for purposes of welfare collection, the failure to consider that this person might be unavailable to make the collection, the decision to provide double authentication only by phone, often inaccessible to claimants, all presuppose a middle-class standard that exacerbates burdens on the most vulnerable.
Similar problems were faced in Brazil after the implementation of facial recognition systems in public buses to verify users’ identity and prevent third parties from using the free card passes granted to children under five years of age and people with disabilities, or the scholar passes given to students. The system is designed to block the card if it cannot match the person with the ID. In some cities, the system fails to identify children with disabilities, as the height of the facial recognition camera prevents them from reaching it, resulting in unfairly blocked ID cards which can only be unlocked upon payment of a fee. Profiling and discrimination, surveillance and control, targeted scams, and advertisements are other underlying risks of datafied public policies.
2. Social protection and privacy
As these examples indicate, social protection systems across the world are becoming increasingly computerized and reliant on beneficiary data for decision-making (Masiero and Das, 2019). This is especially so when such programs rely on focalization. The promise of datafication of social programs lay in being able identify and include (with some precision) those who need it, and exclude the ones who don’t. For this very reason, the United Nations identified in the use of data a revolutionary potential that could accelerate the journey towards sustainable development.
But while it is eminently possible for governments to adhere to principles of inclusion and justice in the use of digital technologies, these are often adopted without proper considerations of risks to privacy, autonomy, and equality. Algorithmic decision-making is prone to errors, and decisions around the adoption of technologies can result in unfair exclusions. Especially in societies characterized by extreme inequalities – inevitably reflected in the data collected on their members – the management of information and its use for decision-making must be under intense scrutiny, review, criticism, and social control.
It is not only a matter of discrimination resulting from the datafication, but discrimination in datafication. The technology is being incorporated first and foremost in programs and facilities that carry out public policies, and in a non-optional way. That is, the collection and processing of data are inevitable when citizens access public services and exercise their rights.
This leads to associated privacy concerns. Privacy, besides leading to autonomy and self-determination and being a right on its own, it is also a precondition for other rights such as freedom of expression and of association and assembly. It is, therefore, central to political participation. However, the burden of exercising such rights lays heavier on some communities than others, for instance, women and people of color. For this reason, their exposure to risks associated with data can cause more harm.
In 2019, the UN Rapporteur on Extreme Poverty dedicated their annual report to social protection and digital technologies. The report presents several case studies showing how, in the name of fraud detection, savings, and efficiency, citizens are obliged to give up on privacy, autonomy, choice, and dignity. These problems are unfolding both in the Global North and South, and can be summarized as follows: digital technologies offer an irresistible promise because of how they can tackle fraud and eliminate friction in the awarding of benefits; however, their shortcomings and potentially negative impacts and consequences are underestimated, and the causes they serve end up with sub-optimal results in the process.
In a situation of economic deprivation, one can hardly refuse to disclose data, especially if the access to assistance depends on such disclosure. Because refusal is not plausible and consent is either not required or effectively not free, these programs must embed privacy and data protection in their legal and technical design.
Individuals are not in a position to resist these processes. In a situation of economic deprivation, one can hardly refuse to disclose data, especially if the access to assistance depends on such disclosure. Because refusal is not plausible and consent is either not required or effectively not free, these programs must embed privacy and data protection in their legal and technical design. It is in this sense that when conceiving social protection programs, it is not enough to think of privacy as a negative right – by asking the state to abstain from the individual private sphere. Data enables and informs social assistance; and the damages caused by unfair processing of data are collective and require collective legal protection.
This means that data processing operations must go beyond compliance with principles such as lawfulness, fairness, transparency, purpose limitation, data minimization, accuracy, security, and accountability. They must also reaffirm the fundamental purposes of social protection, that is, ensuring dignity in the face of risks arising from a market economy. Social protection with unprotected data deepens vulnerability and aggravates inequality instead of solving it.
In the overarching analysis, when combined with austerity policies and their links to social protection and state expenditure, the deployment of extensive data collection and processing operations may serve predominantly to exclude beneficiaries and focus less on identifying vulnerabilities. But technology and data can serve better ends. For instance, they can be used to identify sectors where there is an “assistential vacuum” so that public policies can be directed accordingly. To allow for these questions to be seen and debated, governments must strip the use of technologies from techno-determinist assumptions3 and use a proper and transparent framework for their design and deployment. The data justice dimensions developed by existing literature are extremely helpful in this regard.
3. Data justice and the case of social protection
First of all, data justice is a call for bringing justice first. The distinctions between online and offline, analogical and digital, are becoming increasingly blurred, as analysts from different fields weigh in on these debates. Choices about procedures to collect and treat data, algorithmic decision-making, which systems to contract, and who the subjects of these systems and processes may be, are political decisions. The benefits and potentials of data should be highlighted with this perspective in view.
Second, social protection programs need to rely on diagnoses of how data systems discriminate or perpetuate inequalities, as well as discipline and control. This essay has highlighted a few such instances but more research and dialogue on this is welcome. There are a few academics, research centers and civil society organizations promoting these ties. Some examples are Fundación Karisma in Colombia, Derechos Digitales in Chile, Privacy International, the Digital Welfare State and Human Rights Project at the Center for Human Rights and Global Justice at NYU School of Law, the Research Group for Information Systems at the University of Oslo, and the Global Data Justice project at Tilburg University.
Linnet Taylor proposes a critical discussion on how a rights-based approach might not be the most appropriate to develop a transnational framework for data justice. Such a framework is needed urgently as citizens in specific jurisdictions suffer specific impacts because their data is treated somewhere else – especially when public-partnerships are at stake and transnational corporations play a role in the production or processing of data about citizens. National legislation is unable to address some of the challenges posed by these data processes. Different societies may care about privacy, for instance, without formally recognizing it as an individual right.
A rights-based approach should, therefore, be replaced by a collective and needs-based approach; and this should be accompanied by relevant international frameworks and legislations. Besides transnational solutions, data justice frameworks provide inputs for immediate consideration at the national level as well. Taylor proposes a framework based on three pillars: a) visibility (the need to be represented but also to opt out of data collection and processing); b) digital (dis)engagement (relating to the need to preserve autonomy and sharing data’s benefits), and c) countering data-driven discrimination. This framework goes far beyond privacy and the existing international frameworks for data protection, such as the OECD’s Fair Information Principles – although privacy is an important factor.
All these pillars involve difficult questions, as Taylor recognizes. The integration between visibility, fair representation, and autonomy is hard to reach. For instance, should people be allowed to opt out of the census, which is, despite the confidentiality of the information, an invasive moment in the relationship between the individual and the state and, at the same time, an essential part of citizenship in democracies?
Policy-making processes should be open, inclusive, and participatory, and account for procedural justice, which is an important part of data justice. Human rights concerns must be built into the decision-making process for a new social program or reform of an existing one.
Ultimately, policy-making processes should be open, inclusive, and participatory, and account for procedural justice, which is an important part of data justice. Human rights concerns must be built into the decision-making process for a new social program or reform of an existing one. Privacy, security, and data protection must be part of the standards that need to be met. Differences between contexts and legal frameworks considered, systems should be secure, audited, accountable, and transparent to citizens. They must also guarantee that human intervention is easily accessible whenever errors are identified. The dignity of beneficiaries should be paramount in data sharing, which should, in turn, be limited to what is strictly necessary.
Notes
- 1 Valente, Fragoso and Neris, upcoming (2020).
- 2 Other recent measures in Brazil raise the bar on the concerns around maximalist data collection, sharing and treatment. Decree 10.047 / 2019 included the Single Registry among other 51 databases free for access for the National Institute for Social Services; Decree 10.046/2019 created the so-called Base Register (“Cadastro Base”) to consolidate federal databases and “biographical attributes”, including biometric, and facilitate data sharing between public offices. This Decree has been widely criticized by data protection and privacy researchers and advocates, including ourselves.
- 3 Techno-determinism or technological determinism is the assumption that social changes can be explained by technological advances only, or primarily. It refers to giving undue weight to technology in certain processes. Although it is mostly used for analytical purposes, we here refer to policy decisions that promote the adoption of technologies aiming at producing social and economic results, without taking other factors into consideration, which will influence how these technologies are appropriated or the effects they will cause.
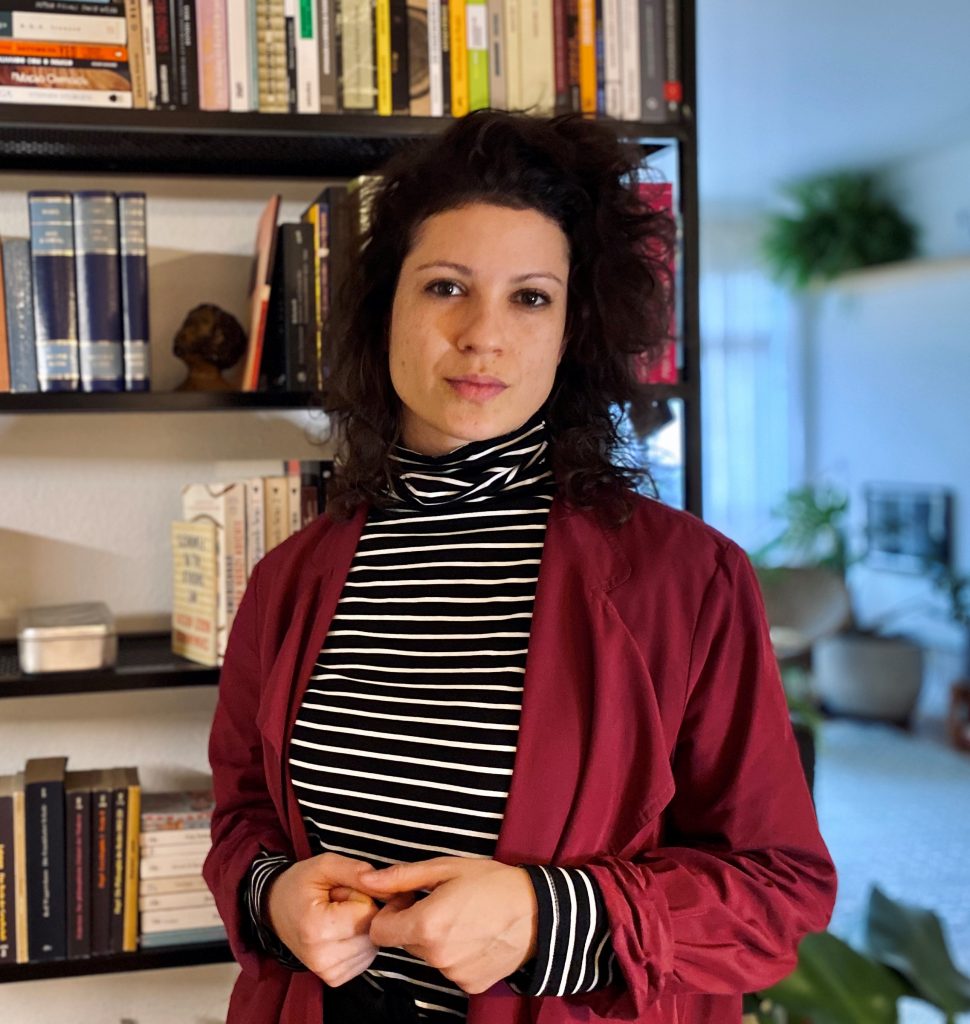
Mariana Valente is the Director of InternetLab (Brazil) and Professor at Insper University, São Paulo. She has a PhD in Sociology of Law from the University of São Paulo, where she also earned her Bachelor’s and Master’s degree. She is also a former visiting researcher at the Berkeley School of Law.
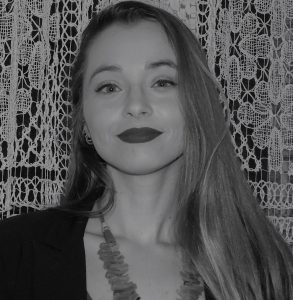
Nathalie Fragoso is the head of research on privacy and surveillance at InternetLab (Brazil). She has a PhD in Sociology of Law from the University of São Paulo Law School, where she also earned her Bachelor’s degree. She has also an LLM (Master of Laws) from the Ludwig-Maximilians-Universität München.